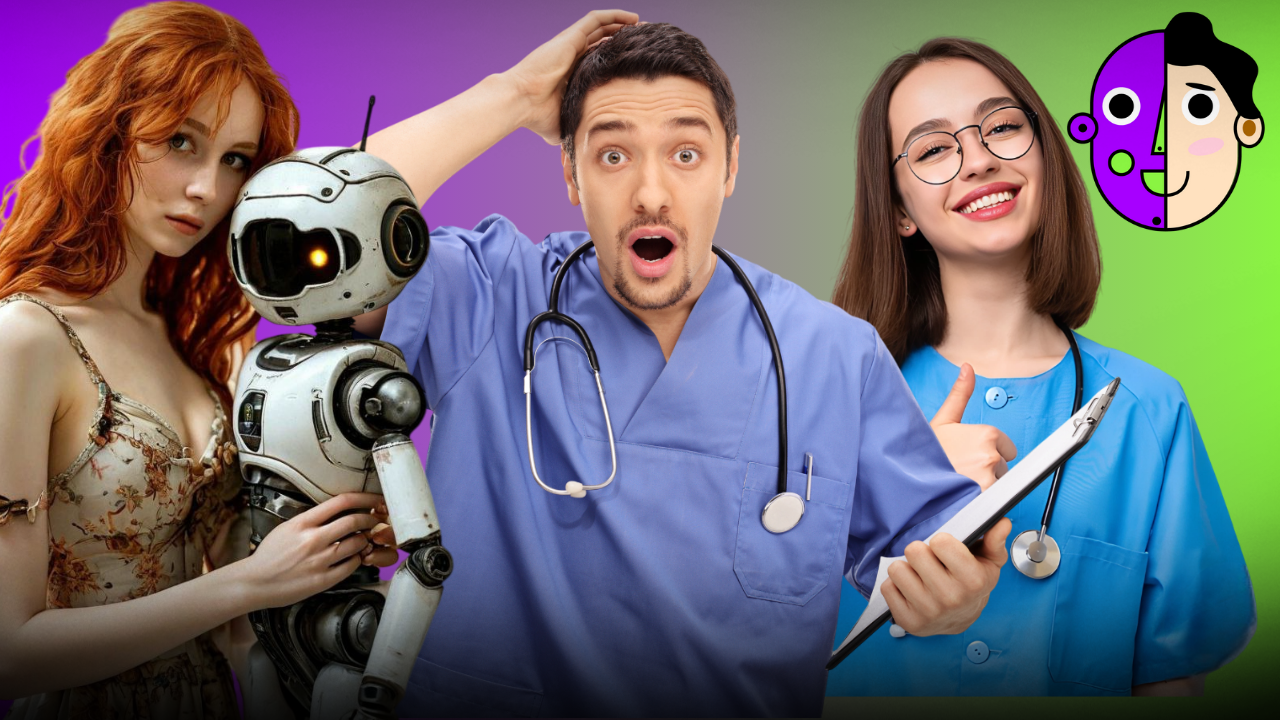
AI in medicine: Breakthroughs doctors don’t talk about
The transformation of medicine through artificial intelligence isn’t just a technological leap. It’s a fundamental change in the approach to treatment and diagnosis of diseases. Research shows that the global artificial intelligence market in healthcare will grow to one hundred and forty-five billion dollars by the thirtieth year. To understand this growth – in twenty-four, this market was thirty billion dollars. That’s almost a fivefold increase in six years! Let’s figure out what’s behind these numbers.
Artificial intelligence technologies in medicine solve several key problems. They improve diagnostic accuracy, accelerate drug development, and make treatment more personalized. Deep learning algorithms can analyze medical images with accuracy exceeding experienced radiologists by fifteen to twenty percent in detecting certain pathologies. This doesn’t replace the doctor but gives them a powerful decision support tool. In drug development, artificial intelligence reduces the journey from idea to finished product from fifteen years to five years, meaning faster patient access to new treatments.
What does this mean for the average person? In the coming years, you’ll be able to get a more accurate diagnosis, more effective treatment, and doctors will spend more time directly working with patients instead of paperwork. Predictive models will help prevent disease before symptoms appear, based on your genetics and lifestyle.
The doctor of the future must be able to work in tandem with intelligent systems. Data interpretation skills and critical thinking will become no less important than traditional medical knowledge. Next, I’ll examine companies and what they’re doing to connect a traditional industry like medicine with a new technology – artificial intelligence.
Medical imaging
The story of Butterfly Network began in eleven when scientist-entrepreneur Jonathan Rothberg founded a startup with an ambitious goal. To create a new portable medical imaging device that could make both magnetic resonance imaging and ultrasound studies significantly cheaper and more efficient. And to automate much of the medical imaging process.
Medical imaging is a set of technologies that allow doctors to “look” inside the body without surgical intervention. These methods include X-ray, ultrasound, computed tomography, MRI, and others. Each of which shows internal organs and tissues in its own way. These studies help doctors detect diseases, injuries, or abnormalities that cannot be seen with the naked eye. Thanks to medical imaging, one can not only make an accurate diagnosis but also monitor treatment effectiveness.
Let’s look at the global picture: about four billion seven hundred million people worldwide don’t have access to medical imaging. From underserved communities in developed countries to remote areas of Africa. Diagnostic capabilities that we take for granted remain inaccessible to most of humanity.
The first step toward achieving this goal was the Butterfly iQ device. This portable system uses revolutionary Ultrasound-on-Chip technology, which replaces the traditional sensor system with a single silicon chip. What does this mean in practice? Conventional ultrasound machines use piezoelectric crystals arranged in certain configurations to achieve the desired depth and type of examination. Different types of examinations require different sensors. Linear ones for surface structures, convex for examining internal organs of the abdominal cavity, phased for cardiological examinations.
Butterfly Network’s engineers completely rethought this technology. They integrated thousands of transforming elements directly onto the circuits that control them, at the semiconductor wafer level. This allowed packing enormous computing power into a chip the size of a postage stamp. Eliminating the need for piezoelectric crystals – which became a revolutionary change in the industry.
The technology of capacitive micromechanical ultrasound transducers provides imaging capabilities equivalent to expensive stationary systems within a single portable sensor for the entire body. Butterfly devices are equipped with more than nine thousand silicate elements that vibrate to generate and receive sound waves, providing a wider bandwidth than traditional ultrasound systems.
This innovative design allows combining phased, convex, and linear sensors in one device without compromising image quality, while simultaneously including advanced imaging capabilities typically found in high-end stationary systems.
But Butterfly is not just a portable ultrasound sensor. The company has created a holistic ecosystem that combines semiconductors, artificial intelligence, and cloud technologies. Whole-body sensors integrate with advanced artificial intelligence, workflow management software, and a comprehensive educational package. Providing a complete solution for ultrasound diagnostics at the point of care, tailored to meet the specific needs of physicians.
Thanks to the combination of semiconductors, artificial intelligence, and cloud technologies, hundreds of thousands of customers worldwide use Butterfly to improve healthcare quality. This technology makes remote medical imaging a reality, which is a blessing for remote communities, some of which are gaining access to such important medical information for the first time.
In individual physician practices, they allow quick primary diagnostics right in the office without referring the patient to a separate department. In large medical systems, Butterfly integrates into the overall care platform, providing a single diagnostic standard across all units. In medical education, the devices allow students to practice ultrasound diagnostics with much lower costs.
The application of Butterfly in resource-limited settings is particularly important. A sturdy, mobile, life-saving device becomes indispensable in humanitarian missions and emergencies. Imagine a situation: in a remote village, a person complains of severe abdominal pain. Without access to diagnostic equipment, the local healthcare worker could only guess the cause. And possibly refer the patient to a district hospital, requiring long and complicated transportation. With Butterfly, the same healthcare worker can immediately conduct a basic ultrasound examination, identify appendicitis, for example, and make an informed decision about the need for urgent evacuation.
Behind these innovations is a solid scientific foundation: the company owns more than six hundred patents. And progress doesn’t stop. With each new generation of Butterfly devices, image quality improves, functional capabilities expand, and artificial intelligence algorithms are refined.
In the future, we can expect further integration of artificial intelligence for automatic interpretation of ultrasound images, which will allow even healthcare workers with minimal training to obtain clinically significant information. Telemedicine functions are also likely to develop, when a specialist from another part of the world can remotely control the examination process and interpret the results.
For patients, this means faster diagnosis, reduced waiting times, and less need to move between different medical facilities. In rural and remote areas, this can mean the difference between timely help and serious complications.
For healthcare systems, the introduction of such devices can lead to more efficient use of resources. Basic diagnostics can be performed at the primary level, referring only those patients who truly need it for more complex and expensive examinations.
For doctors and medical workers, Butterfly provides a tool for making more informed clinical decisions right at the patient’s bedside. This is especially important in emergency situations when minutes count.
Drug development process
The traditional drug development process is a long, expensive, and extremely inefficient journey. From discovering a potential compound to its market release takes an average of fifteen years. And the cost of developing a new drug can exceed a billion dollars. Most drug candidates fail in late-stage clinical trials due to unforeseen side effects or insufficient effectiveness.
The main problem lies in the enormous number of possible biological targets and chemical compounds that need to be tested. The human body contains about twenty thousand genes and hundreds of thousands of proteins. And the number of potential small molecules for drugs is in the billions. It’s simply impossible to check all these combinations with traditional methods. This is where Recursion comes in. Founded in thirteen, the company set an ambitious goal. To create a fundamentally different platform for drug discovery, combining advanced elements of high-throughput biology and automation with the latest advances in artificial intelligence.
Recursion sometimes calls its approach a transition from Bio Tech to Tech Bio. Which reflects a fundamental change in thinking. Instead of starting with biology and looking for technological solutions to specific problems, the company starts by building a powerful technological platform capable of processing biological data at unprecedented scales.
At the core of Recursion’s approach is the creation of enormous arrays of biological data suitable for analysis using artificial intelligence. To date, the company has photographed tens of billions of human cells. And generated over nineteen petabytes of biological data to train its algorithms. To understand the scale: one petabyte is a million gigabytes.
Combining computer vision with classical machine learning and neural networks, the company can conduct about two million experiments every week. That’s about a thousand times more than possible in traditional laboratories. This approach allows Recursion’s algorithms to identify new drug candidates, mechanisms of action, and potential toxicity, which can lead to the creation of new treatments for patients.
In twenty-four, Recursion completed construction of BioHive two – an extremely powerful supercomputer. Designed to accelerate the discovery of new drugs using advanced artificial intelligence and extensive biological datasets. This supercomputer allows processing and analyzing petabytes of data. Modeling complex biological systems and conducting virtual experiments at scales impossible for traditional research groups.
Recursion’s automated laboratories, operating on this supercomputer, collect, model, and analyze target data and perform experiments using computer modeling. The company’s machine learning models identify the most promising targets and are constantly and exponentially improving.
Another important aspect of Recursion’s work is applying artificial intelligence to create new molecules. Using generative artificial intelligence, predictive models, and experimentation, the company develops, synthesizes, and tests new molecules. Optimized for efficiency, selectivity, safety, and bioavailability.
Unlike the traditional approach, where chemists manually design and optimize molecules, Recursion uses algorithms capable of predicting the properties of millions of potential compounds. And offering the most promising candidates for synthesis and testing. This significantly reduces the time and resources needed to find molecules with desired properties.
One of Recursion’s most interesting innovations is LOWE. They named this language agent based on a large language model, which allows scientists and technologists to directly interact with Recursion’s operating system. With it, researchers can identify new targets, generate new compounds, and even plan synthesis and experimentation with these compounds.
LOWE, which stands for “Language-model Orchestrated Workflow Environment,” represents the next evolution of Recursion’s operating system. It supports drug discovery programs by coordinating complex workflows. These processes combine various stages and tools, from finding meaningful relationships in Recursion’s biology and chemistry maps to generating new compounds and planning their synthesis and experiments.
Traditionally, early stages of drug discovery involve multidisciplinary collaboration between teams of chemists and biologists over several months or years. Within Recursion, this process typically requires biologists to define biological pathways and establish new connections on the map, and then chemists to optimize chemical series for selected targets.
LOWE simplifies these processes by combining these disparate functions into a single user interface that works using natural language commands. The growing number of artificial intelligence tools being developed and datasets being generated at Recursion increases the complexity of early drug discovery workflows.
Accelerating the drug discovery process can lead to new treatments for diseases for which there are currently no effective drugs. From rare genetic disorders to antibiotic-resistant infections. Many medical problems remain unsolved due to the complexity and cost of the traditional drug development process.
Additionally, Recursion’s approach can reduce the overall cost of drug development, potentially leading to more affordable medications for patients.
And using artificial intelligence can help identify new applications for existing drugs, known as drug repurposing. This can be particularly valuable for quickly responding to new health threats. For example, such as pandemics.
Brain-computer interfaces
Now, into the medical field bursts Elon Musk and his Neuralink project. Which works in one of the most exciting areas at the intersection of neuroscience and technology – brain-computer interfaces.
Let’s start by understanding what a brain-computer interface is. Essentially, it’s a technology that creates a direct communication channel between the brain and an external device. Allowing control of computers, prostheses, or other devices by “thought” without the need for movement. Such interfaces open up enormous possibilities both in medicine for people with disabilities and for potential enhancement of human abilities in the future.
Neurointerfaces have been researched for decades, but most existing solutions have serious limitations. Non-invasive devices positioned on the head surface cannot accurately read signals from individual neurons. Traditional invasive solutions are often bulky, require wired connections, limit patient mobility, and carry high risks of complications.
In this context, Neuralink was founded in sixteen. Created by Elon Musk and a group of eight scientists and engineers, the company was first publicly presented in March seventeen. Interestingly, the name “Neuralink” and the prototype on which the company began its work initially belonged to two neuroscientists – Pedram Mohseni and Randolph Nudo. These researchers were developing an electronic chip to treat traumatic brain injuries but couldn’t get enough investor support to continue their work.
Neuralink’s official mission sounds ambitious: to create a universal interface for the brain. To restore autonomy to people with unmet medical needs today and unlock human potential tomorrow.
What distinguishes Neuralink among other developments in this field? Besides the world-famous founder, of course. First and foremost, it’s a unique approach to creating an invasive neurointerface that solves many problems of existing technologies.
In nineteen, Neuralink presented a device resembling a “sewing machine.” Capable of implanting very thin threads four to six micrometers thick into the brain. For comparison, a human hair is about seventy micrometers thick, so these threads are approximately fifteen times thinner. At the same time, a system was demonstrated that reads information from a laboratory rat through one thousand five hundred electrodes.
The current N one implant from Neuralink is a fully implantable device. Cosmetically invisible and designed to control a computer or mobile device anywhere. It records neural activity through one thousand twenty-four electrodes distributed across sixty-four threads. These highly flexible, ultra-thin threads are key to minimizing damage during implantation and thereafter.
The implant is hermetically sealed in a biocompatible housing that withstands physiological conditions several times harsher than in the human body. It’s powered by a small battery, charged wirelessly from outside using a compact inductive charger, making it easy to use anywhere. Advanced, specially designed energy-efficient chips and electronics process neural signals. Transmitting them wirelessly to the Neuralink application, which decodes the data stream into actions and intentions.
One of Neuralink’s most impressive innovations is the surgical robot designed for implant insertion. The implant threads are so thin that they cannot be inserted by human hand. The surgical robot was developed for reliable and efficient insertion of these threads precisely where they need to be.
The robot consists of a base structure and movement platform that provide the structural foundation for the robot head. And the main three-axis linear movement used to position the robot head and needle. The robot head contains optics and sensors of five camera systems and optics for the optical coherence tomography system. The needle, thinner than a human hair, captures, inserts, and releases threads.
Neuralink’s journey from laboratory development to real patients hasn’t been the smoothest. The company has faced criticism due to the large number of primates that were euthanized after medical trials. Veterinary records showed complications with surgically implanted electrodes in monkeys. Nevertheless, in May twenty-three, the company received approval to conduct human trials in the US.
And on January twenty-ninth, twenty-four, Elon Musk announced that Neuralink had successfully implanted a device in a human, and the patient was recovering. This became an important milestone for the company and the entire neurointerface industry. Even more impressive is the company’s announcement in September twenty-four that its latest development, Blindsight, will allow blind people with undamaged visual cortex to regain some level of vision. This development received “breakthrough” status from the US federal government, which will accelerate its development.
Let’s now look at the potential applications of Neuralink technology and similar neurointerfaces in medicine and beyond.
First and foremost, brain-computer interfaces have enormous potential for people with paralysis. They can allow such patients to control computers, wheelchairs, prostheses, and other devices directly with thought, substantially increasing their autonomy and quality of life.
As we’ve already mentioned, the technology can also help blind people with intact visual cortex restore vision. This works by directly stimulating the visual cortex of the brain, bypassing damaged eyes or optic nerves.
Additionally, neurointerfaces can find application in treating various neurological disorders such as epilepsy, Parkinson’s disease, depression, and post-traumatic stress disorder by monitoring and modulating brain activity.
In a more distant perspective, such technologies can be used to enhance cognitive abilities. Improve memory, learning, and even create new forms of communication between people, but this borders on science fiction and requires solving many technical, ethical, and social questions.
But it’s necessary to note that many serious challenges stand in the way of developing neurointerface technologies. From a technical perspective, it’s necessary to ensure long-term stability and safety of implants, improve the accuracy of decoding neural signals, and minimize the body’s immune response to a foreign object.
From an ethical perspective, questions arise about the privacy of neural data, potential hacker vulnerability of implants, social inequality in access to such technologies. And even fundamental questions about how these technologies might change our understanding of human personality, free will, and consciousness.
Healthcare map
Modern healthcare generates colossal volumes of data. Every doctor visit, every test, every prescription – all of this forms a huge array of information. Which theoretically can give us invaluable knowledge about disease progression, treatment effectiveness, and much more.
However, traditionally this data remains fragmented, hard to access, and difficult to analyze. According to a Frost & Sullivan study conducted in twenty-four, healthcare companies spend an average of seven months just to prepare data in a usable state. Imagine: half a year of preparation before you can even begin to analyze the information!
In this context, Komodo Health, founded in fourteen by Web Sun and Dr. Arif Nathoo, set out to solve this fundamental problem. Initially, the company set an ambitious goal – to create the most complete and detailed healthcare map in the world. That would track so-called “patient journeys” through various medical facilities and types of treatment.
Over ten years, Komodo Health has transformed into one of the leading companies in applying artificial intelligence in healthcare. And the result of this development was the revolutionary MapAI platform, which processes information on more than three hundred thirty million patient histories. This platform combines artificial intelligence with the most complete healthcare map in the industry. To provide real-time analytics on disease trends, treatment pathways, and patient groups.
The key innovation of Komodo Health’s latest platform, called MapLab, is an integrated analytics assistant based on artificial intelligence. Which allows users to generate analytical insights using natural language processing. In other words, now any person, even without technical knowledge, can simply ask a question in ordinary words and receive an answer based on the analysis of millions of medical cases.
Now let’s look at how MapAI is applied in various areas of healthcare and pharmaceuticals.
In clinical development, teams can use MapAI to study the disease landscape, identify medical organizations and workers treating certain patient groups. And determine the impact of inclusion and exclusion criteria for clinical trials.
In the field of medical communications, MapAI accelerates strategic tasks. Specialists can start with a general disease analysis, improve physician engagement strategies, and measure the real impact of educational and informational programs.
In the commercial sphere, MapAI helps accelerate understanding of the market landscape, conduct segmentation of patients and healthcare workers, track the effectiveness of pharmaceutical brand promotion, and much more.
Essentially, MapAI works on a principle similar to ChatGPT, but specializes specifically in medical data and questions. And the introduction of tools like MapAI can have far-reaching consequences for the entire healthcare industry. Let’s consider some of them.
First, a significant reduction in time from question to answer. Instead of months collecting and preparing data, researchers and analysts can get answers to complex questions in minutes or hours. This can significantly accelerate research on new drugs, optimize treatment, and respond to new health threats.
Second, democratization of access to medical data. Now not only data specialists but also doctors, researchers, clinic and pharmaceutical company managers can directly work with information and get valuable insights. This can lead to more informed decisions at all levels of the healthcare system.
Third, the ability to see the complete picture. By combining data from various sources and tracing the “patient journey” through the entire healthcare system, tools like MapAI allow seeing general trends and patterns that might remain unnoticed with a more fragmented approach.
It can be expected that in the future, similar tools will be even more integrated into all aspects of healthcare, from drug development to individual patient treatment. With the increase in volume and quality of data, as well as improvement of artificial intelligence algorithms, the accuracy and usefulness of such tools will only grow.
Smart medical facility
There are such problems that healthcare systems around the world face today. One of the key problems is the shortage of medical personnel with growing demand for medical services. Nurses and other healthcare workers are often overloaded with routine administrative tasks that take away precious time that could be spent on direct patient care.
Another serious problem is patient safety. Patient falls, bedsores, non-compliance with prescribed treatment protocols, and other incidents can significantly worsen treatment outcomes and increase healthcare costs.
Furthermore, traditional monitoring systems often react to events that have already occurred rather than preventing them. This means that medical staff learn about a problem only after it has already arisen, which limits opportunities for timely intervention.
Care.ai developed a comprehensive solution for these problems, creating the concept of a “Smart Care Facility.” This is a platform that works around the clock and without weekends, using artificial intelligence and ambient sensors for continuous monitoring and support of both patients and medical staff.
At the core of the system are intelligent sensors with continuous observation function, installed in patient rooms and throughout the medical facility. These sensors include high-precision cameras and other sensors that continuously collect information about the environment, actions of patients and medical staff.
The collected data is processed using advanced artificial intelligence algorithms. Which can recognize potentially dangerous situations before they arise and automatically notify medical staff. For example, the system can notice that a patient is showing signs of anxiety and may try to get out of bed, creating a fall risk. In this case, the system immediately sends a notification to staff, which allows preventing the incident.
Let’s consider the key components and capabilities of the Smart Care Facility platform from Care.ai.
First, there are Smart Patient Rooms. Thanks to built-in sensors and artificial intelligence, the room becomes a “self-aware” environment. Which constantly monitors the patient’s condition and surrounding environment. The system can track the patient’s position in bed, their movements, signs of anxiety, and other parameters that may indicate potential problems.
Second, there’s an AI-Assisted Command Center. It provides a single interface for monitoring all processes in the medical facility. The command center can work on both stationary computers and mobile devices of medical staff, providing instant notification of emerging problems.
Third, there’s AI-Assisted Virtual Care. This feature allows remote members of the medical team to observe patients and interact with them through screens and cameras built into the sensors. This is especially useful in situations where a specialist’s quick consultation is required, but physical presence is impossible or impractical.
The sensors work based on edge computing technology. Which means that most data processing occurs directly on the device, without the need to transmit large volumes of data over the network.
Now let’s consider what specific problems this system solves and what advantages it provides for medical facilities, staff, and patients.
The first important application is preventing patient falls. The system can detect early signs that a patient is going to get out of bed. Including restless behavior, and immediately notify medical staff. This is critically important, considering that patient falls are one of the most common causes of injuries in hospitals.
The second key application is preventing bedsores. The smart room constantly monitors the patient’s position in bed and can notify staff when it’s necessary to change the patient’s body position to prevent bedsore development.
The third important function is monitoring compliance with medical protocols. The system tracks the execution of prescribed procedures and automatically reminds staff when something doesn’t comply with established norms. This contributes to improving care quality and reducing medical errors.
The fourth application is preventing unauthorized departure of patients from the medical facility. Sensors can track patient movement throughout the facility and notify staff if a patient approaches an exit or crosses an established boundary.
Behind the external simplicity of using the system are complex technological innovations. Let’s consider some of them.
First, there are ambient intelligent sensors with continuous awareness function. These sensors not only collect data but also process it directly on the device using built-in neural networks. This ensures quick system response and reduces the load on the medical facility’s network.
Second, the platform uses federated learning of artificial intelligence. This means that sensors constantly learn and improve, safely sharing acquired knowledge among themselves. Thus, the entire sensor network functions as a single intelligent organism that becomes smarter every day.
Conclusion
Artificial intelligence in medicine is here to stay. Some machine learning systems help doctors make more accurate diagnoses and develop personalized treatment plans. Other medical image analysis technologies allow detecting pathologies at early stages when treatment is most effective. In clinical practice, algorithms process millions of medical records, revealing hidden patterns and predicting disease risks.
These solutions don’t replace doctors but become their assistants, freeing up time for patient interaction. For us as patients, this means better diagnosis, fewer medical errors, and access to advanced treatment methods.
The future of medicine is a symbiosis of human experience and artificial intelligence computational power. Imagine that our medical data is constantly being analyzed, warning about problems before symptoms appear. Sounds cool!