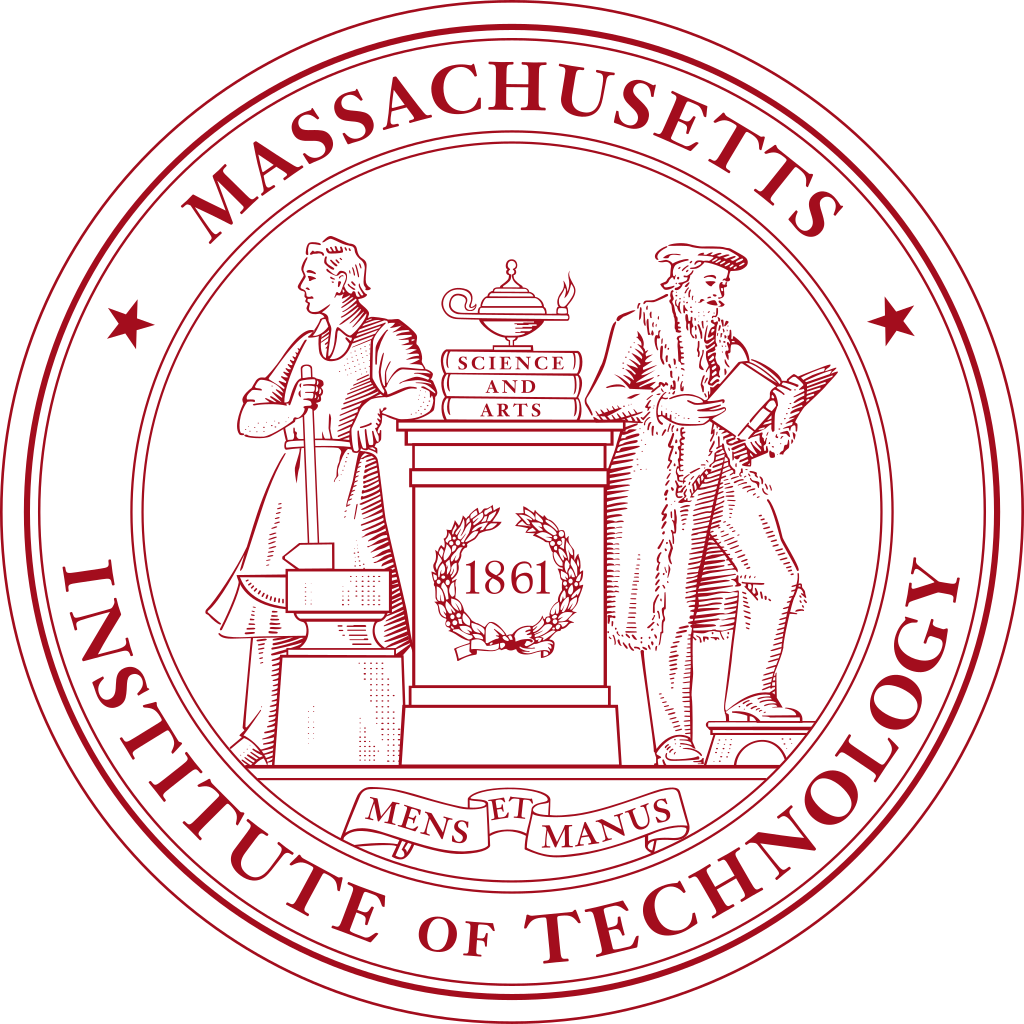
MIT creates breakthrough system for AI model optimization
Researchers at the Massachusetts Institute of Technology (MIT) have presented an innovative automated system that allows radically increasing the efficiency of artificial intelligence models through simultaneous use of two types of data redundancy.
The new development aims to solve one of the key problems of modern deep learning – high energy consumption of AI models when processing complex data structures in applications such as medical image analysis and speech recognition.
Existing algorithm optimization methods typically allow developers to use either sparsity or data symmetry – two different types of redundancy present in deep learning structures. MIT’s innovative approach allows utilizing both types simultaneously, which in experiments led to an increase in computation speed by almost 30 times.
“For a long time, accounting for these data redundancies required significant implementation efforts. Now a scientist can describe the desired computation result to our system in a more abstract way, without specifying the exact algorithm,” explains Willow Ahrens, an MIT postdoc and co-author of the study, which will be presented at the International Symposium on Code Generation and Optimization.
A key advantage of the system is the use of a convenient programming language, making it accessible for a wide range of applications. This is especially important for scientists who are not experts in deep learning but seek to increase the efficiency of AI algorithms in data processing.
MIT’s development opens new perspectives in optimizing computational resources for machine learning, offering:
– Automatic algorithm optimization considering multiple types of redundancy
– Significant reduction in computational power requirements
– Reduction in bandwidth and data storage costs
– Simplified interface for AI system developers
The system also has potential applications in scientific computing, which may contribute to accelerating research in various fields of science where complex computational models are used.