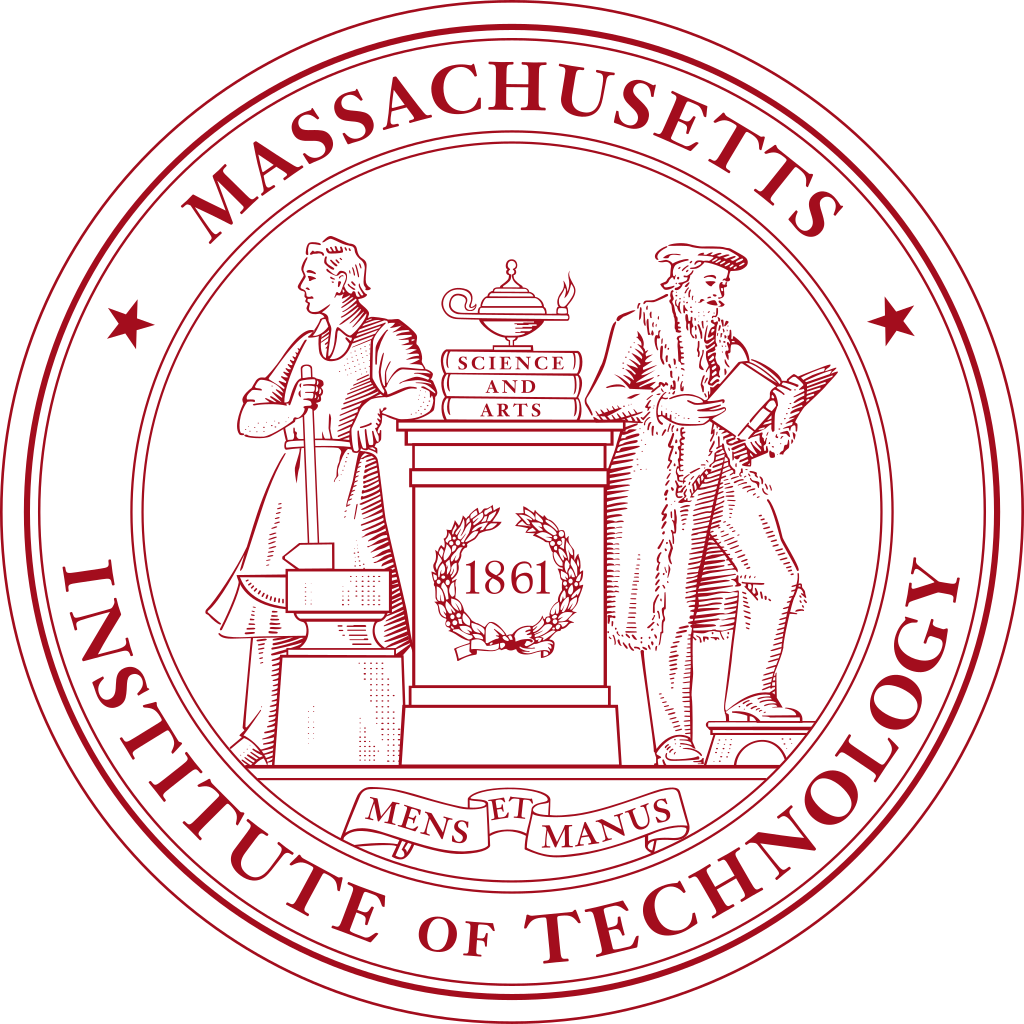
MIT scientists find way to make more accurate predictions
Massachusetts Institute of Technology (MIT) researchers have developed a new method for evaluating spatial predictions that could significantly improve forecast accuracy across various fields – from weather forecasting to air pollution assessment.
The team of scientists discovered that traditional validation methods can produce serious errors when solving spatial prediction problems. As Tamara Broderick, associate professor of electrical engineering and computer science at MIT, explains, the problem lies in incorrect assumptions about the nature of data.
Classical methods assume that validation and testing data are independent and identically distributed. However, this is often not the case in spatial problems. For example, EPA air pollution sensors are placed considering the location of other sensors, and data from urban and rural areas may have different statistical characteristics.
MIT’s new method is based on the assumption of smooth data changes in space. “Air pollution levels are unlikely to change dramatically between two neighboring houses,” Broderick explains. This approach proved more accurate in most experiments, including wind speed forecasting at Chicago’s O’Hare airport and air temperature in five US metropolises.
The technology can find wide application in various fields:
- Climatology (sea surface temperature forecasting)
- Epidemiology (assessing air pollution’s impact on diseases)
- Meteorology (weather forecasting)
- Environmental monitoring
The research, supported by the National Science Foundation and Office of Naval Research, will be presented at the International Conference on Artificial Intelligence and Statistics.